The application of the Internet of Things (IoT) in the industrial sector, known as IIoT (Industrial Internet of Things), has revolutionized communication and operation between manufacturing devices. In manufacturing environments, this technology demands robustness to ensure the reliability and safety intrinsic to production processes.
In this way, the acquisition of data from the physical world is the first step towards the digitalization of industrial processes. Sensors and actuators capture variables such as temperature, pressure, vibration, and position, converting physical phenomena into electrical signals. These signals, often analog, need to be conditioned—amplified, filtered, and isolated—before being digitized by data acquisition (DAQ) systems for further analysis. DAQ systems perform crucial functions such as:
- Signal conditioning, to ensure data integrity.
- Analog-to-Digital Conversion (ADC), transforming continuous signals into discrete data.
- Recording and transmitting data, which can be sent to local servers or cloud platforms.
The typical architecture of an IIoT network is structured in layers, the first being perception, in which sensors monitor variables such as temperature, pressure, and energy consumption. A second, the network layer, is responsible for transmitting this data through technologies such as LAN, WAN, Wi-Fi, and 5G. Next, the analysis layer processes the information, either locally (edge computing) or in the cloud (cloud computing), to identify patterns, predict failures, and optimize processes. Finally, the application layer uses these insights for informed decision-making and task automation.
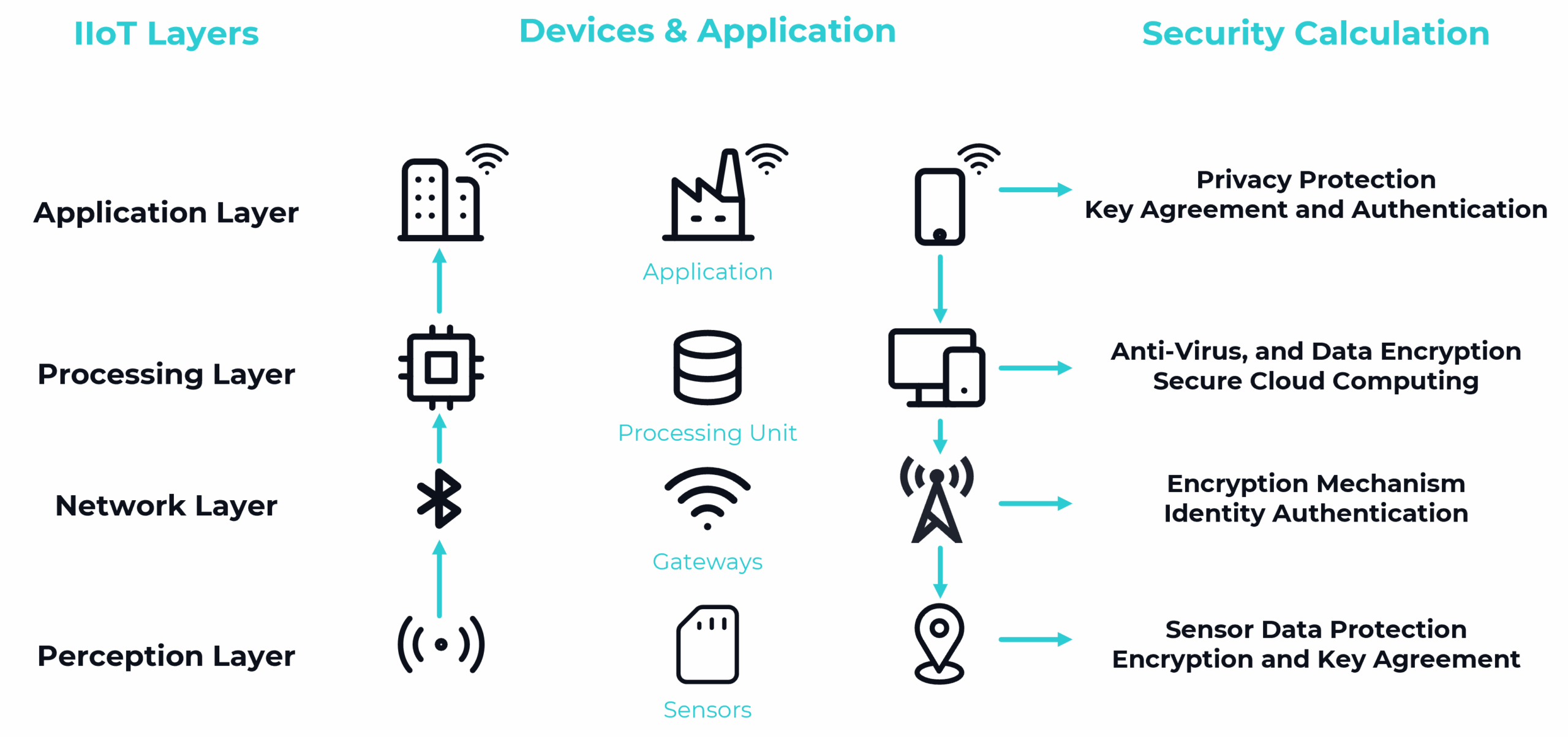
Another significant benefit of IIoT lies in its ability to promote interoperability between machines operating with different protocols and architectures, facilitating the integration of complex systems.
Communication protocols in the IIoT
The IIoT infrastructure enables real-time data collection, which contributes to optimizing the reliability and efficiency of the entire connected system. For transmission between sensors to be effective, there are different communication protocols, each designed to meet particular needs. The operating frequency, the IEEE (Institute of Electrical and Electronics Engineers) standard, and the transmission range are all criteria to describe them. Some of them are:
Protocol | Interoperability | Performance (Data Rate, Latency, Real-Time) | Security | Scalability | Value for Money |
Modbus | Originally designed as a serial protocol, but there are versions that operate over ethernet infrastructure | Lower bandwidth. It can be slow, especially with serial connections. | It usually depends on the security of the network. | Limited scalability for large installations. | Low hardware cost. |
PROFINET | Utilizes standard Ethernet technology. | Real-time communication capabilities. High data rates. | Supports secure and efficient data transmission. | Highly versatile with multiple topologies. | May involve costs for specialized cables, Ethernet switches, and interface modules. |
OPC UA | Platform-agnostic standard for OT/IT integration. | Supports complex data types, events, and alarms. | Robust data modeling, certificate-based encryption. | Highly scalable. | Initial implementation can be costly, such as with development toolkits (SDKs) and appropriate hardware resources. |
MQTT | Lightweight signing protocol. Native support on major cloud platforms. | Low bandwidth usage. Higher latency compared to some industrial Ethernet protocols. | Relies on underlying protocols like SSL/TLS for security. | Highly scalable. | Requires a broker to manage message routing. It may require development resources, such as for the need for coding to interpret payloads. |
LoRaWAN | Long-range communication. It operates in bands of the radio spectrum. | Suitable for low power consumption and long range applications. Latency is typically seconds. | AES-128 encryption for data security. | Scalable, supports massive device connectivity. | Low cost for wide area coverage. |
IoT Data Storage Strategies in Industry
After acquisition and transmission, IIoT data needs to be stored in robust infrastructures. The three most relevant approaches in this context are cloud computing, edge computing, and temporal databases.
Cloud computing offers a scalable and flexible solution for storing and processing large volumes of data. Platforms such as AWS IoT, Azure IoT Hub, and Google Cloud IoT integrate advanced real-time analytics and visualization capabilities. This approach is ideal for applications that require historical analytics, machine learning models, and integration with enterprise systems.
Complementing this strategy, edge computing processes data close to the source — such as in industrial gateways or local controllers — reducing latency and network traffic. This feature is crucial in scenarios that demand a quick response, such as real-time process control or predictive maintenance. In this approach, only the most relevant data is sent to the cloud, optimizing the use of bandwidth and storage resources.
Time-series databases (TSDBs) are aimed at storing time series, that is, data associated with timestamps. They allow you to follow the evolution of variables over time, being ideal for data that arrives continuously and at high frequency. In addition, they enable queries by time intervals, facilitating trend analysis, anomaly detection, and data comparison. To ensure performance and space savings, they use efficient compression techniques, taking advantage of repetitive and predictable patterns in the data.
These three approaches, combined, form a solid foundation for efficient and intelligent data management in the industrial environment.
Application of IoT in different industry sectors
According to IBGE (2023), 84.9% of medium and large industries in Brazil already use some advanced digital technology, with IoT being an important part of this scenario. The benefits provided by IoT in areas such as production and operations are evident, which explains the concentration of its applications in these sectors. This finding is reinforced by the IoT Snapshot (2022) study, conducted by Logicalis, which reveals that more than 60% of the companies surveyed have IoT solutions implemented, in the pilot phase, or in proof of concept in these same areas. In a global context, the IoT market in manufacturing industries presents significant potential, with projections by Fortune Business Insights (2024) indicating a reach of $452.27 billion by 2032.
IIoT technology demonstrates its versatility and impact in several industrial sectors: in the automotive sector, it optimizes internal logistics and component tracking, streamlining processes such as recalls, and drives investments in production optimization and predictive maintenance, with about 30% of Brazilian industries in the sector engaged in this transformation; in the food and beverage industry, IoT enables production and quality monitoring, ensuring compliance with standards and consistency of products such as beer, in addition to promoting efficiency in the use of utilities such as water and energy, aligning with ESG practices; Finally, in the pharmaceutical and chemical sector, continuous monitoring of production and storage conditions, especially temperature, ensures product integrity, while the consistency of indicators in chemical reactions reinforces operational safety.
According to IDC, by the end of 2025 there will be more than 55 billion connected IoT devices globally, generating about 80 zettabytes of data. This exponential growth drives organizations to expand their digital ecosystems, integrating internal processes and establishing strategic partners, with the aim of increasing resilience and operational flexibility. In addition, the convergence of IoT with technologies such as machine learning and advanced analytics enables autonomous operations and real-time decisions.
Explore how best to collect data, even under harsh conditions with the ST-Spots line™.
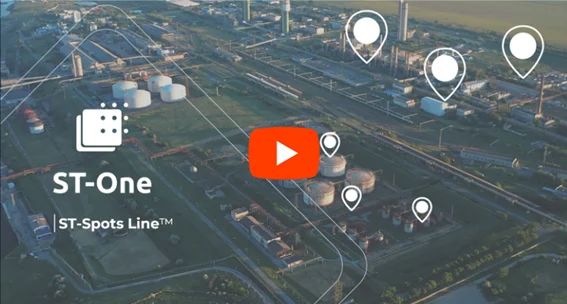
View on AR on your phone: